In recent years, health technology policy-making science has gone beyond just a health technology assessment or systematic review or economic evaluation study and the science of operational research in decision making, i.e. multi-attributes and multi-objective decision-making has been included. Hence, currently, health technology policy-making follows a seven-step process. After a technology undergoes these steps and is proven that it is useful for the health system, it must be determined how many of it is needed in the health system. Determining the required number of health technologies is a challenge that remains to be considered. Therefore, this study was designed to overcome this problem. The authors intend to introduce a multi-objective decision-making methodology considering the limited budget, to determine the number of technologies required to complete for the health technology policymaking cycle.
Key words: Health technologies, Multi-attributes decision-making, Goal programming
Introduction
The health sector is facing resource constraints in both low and high-income countries (1, 2). The consequence of these resource constraints is facing the issue of selection so that resources can be allocated to the possible optimum way. To help for the improvement of this process, methods of prioritizing and how-to of using them are required (3-5). One of the most important ways of prioritizing health services is utilizing health technology assessment in choosing health technologies. Considering that, in recent years the use of medical technologies in the diagnosis and treatment of diseases has grown dramatically, the need for an evidence-based approach for prioritizing and appropriate utilizing the health technologies is crucial. Rational use of these technologies can contribute to the health care efficiency (6).
On the other hand, the unrestricted and uncontrolled providing of these technologies may lead to some challenges, i.e. induced demand and fee-splitting, which will eventually lead to overuse of health services (6
(. This problem has arisen in many developed and developing countries and has led to a sharp increase in costs and inefficiency (7)
. Therefore, before the introduction of technologies, the systematic method is used to evaluate them and allow them to be licensed and utilized to maintain the usage of available sources at an optimal level (6-7). Nowadays, health technology policymaking science has gone beyond just a health technology assessment and the science of operational research in decision making such as multi-attribute decision-making (MADM) and multi-objective decision-making (MODM) has been included. It should be noted that MADM includes techniques that aim to rank the alternatives with respect to decision criteria; while MODM includes the methods that aim to optimize an objective function, taking into account the existing constraints (8). Given to this techniques, new global literature suggests that health technology policymaking can follow seven steps: 1) Installing an advisory committee; 2) Defining decision criteria; 3) Prioritizing research topics for health technology assessment; 4) Scoping (PICOD, standing for Population, Intervention or Index Test, Comparator(s), Outcomes, and Design); 5) Assessment; 6) Appraisal for including health services benefit packages or medical practice; 7) Communication and appeal (9). In the third and the sixth stages, multi-attributes decision-making methods are used (9,10).
Following the technology assessment, the licensing process (technology includes medicine, medical equipment, or services) is facilitated so that the technology can be introduced into the country's health system or included in the health insurance benefit package. If technology goes through seven stages of the health technology policymaking process, it means that it is useful for the health system (9). The only thing left unfulfilled with these seven stages, is that the required number of each technology is not determined which may lead to over-purchasing. The present study was designed to address this challenge and to introduce multi-objective decision making by offering a special methodology and taking budget limitations. This methodology can be used to complete the health technology policymaking cycle and make the output of this process more efficient.
The model that the authors propose is applicable to the sixth step, the technology appraisal stage, to include the health services benefit package or the medical practice to complete this cycle. In fact, this approach can complete the model proposed by Baltussen et al. (11), which introduces decision rules to actualize the results of multi-attributes decision-making models. This study aim to introduce an approach in which at first the most important types of health technologies are identified using MADM techniques, and then the required number of each of them is determined using MODM methods to maximize the values of cost-effectiveness and budget impact attributes. To the best of our knowledge, no one has yet applied MADM and MODM techniques simultaneously to determine the types and the optimal number of each type of health technology to obtain the maximum values of cost-effectiveness and budget impact attributes.
Materials and Methods
In this section, it was tried to present an approach to rank the health technologies and then determine the number of each of the most important types of technologies that should be provided to maximize the values of cost-effectiveness and budget impact attributes (as decision rules). For this purpose, first, the weights of the most important health technologies are extracted from MADM techniques. These weights are then used as coefficients of the importance of technologies in the objective functions of a two-objective goal programming model. This model is an optimization model that includes two separate objective functions; the first and the second ones aim to optimize the values of cost-effectiveness and budget impact attributes, respectively. These objective functions are aggregated and solved through a multi-objective goal programming model. The assumptions, parameters and decision variables, objective functions, and the constraints of the proposed model are described in the Results section.
Results
Assumptions
1. The proposed model is a single-period multi-product model (designed to determine the optimal amount of each technology for a given period).
2. The minimum and maximum demand for each technology is specified.
3. The demand for any technology is certain.
Model indices
i: The type of i
th technology (here we assume n technologies have entered the appraisal stage, so the value of i can vary from 1 to n);
j: The type of index. There are two indices to the goal model. So the value of j can be 1 or 2, which represents the cost-effectiveness and budget impact attributes, respectively.
Model parameters
Ci: The current cost of providing type i technology (purchase cost, shipping cost, etc.)
Wj: The weight of index j (this weight can also be calculated using MADM methods)
Pij: Weight of i
th technology with respect to index j (the weight of each technology with respect to the first index can be obtained either from the exact value of cost-effectiveness of that technology or from the experts' opinion; the weight of each technology with respect to the second index can also be obtained either from the exact value of budget impact of that technology or from the experts' opinion).
B: Total funding for health technologies:
Di (min): the minimum number of i
th technology to be provided;
Di (max): the maximum number of i
th technology to be provided;
Note that the minimum and the maximum number of type i technology to be provided can be estimated using experts' opinion as well as by considering attributes such as the target population size and the availability of alternative technologies.
Decision variables
Xi: Number of type i technology
Objective functions
Maximize Z
1 =
Maximize Z
2 =.JPG)
This research has the following limitations to achieve the above-mentioned goals. These restrictions vary for each period or country. But some of them are as follows:
Type I restrictions: Budget limitation:
In other words, the number of purchases of each technology must be such that the total amount of money paid for them does not exceed the allocated budget.
Type II Restrictions: restrictions to the maximum amount of purchase for each technology:

Type III Restrictions: The restrictions in the minimum of purchase of any type of technology:
In other words, according to experts (healthcare policymakers and technology providers), the minimum amount that any technology should be purchased is defined. This may be determined by social conditions and sensitivities.
The approach to solve the model
In the goal programming method, the optimal value is first obtained for each of the indices separately and by solving the individual optimization models; then, a goal programming model is designed, which has two more goal limitations in addition to the previous limitations. The objective function associated with each index is equal to the optimal value of that index and is added to the model as a goal limitation. So, there are two goal limitations as follows:
The value of

represents the optimal value of goal j, which is obtained by solving the separate models.

and

are also the desired and undesirable deviations from the j
th goal. As it is clear, the variables of

are undesirable deviations (provided all the goals are max) and should be minimized. But these variables have different scales, so they have to be normalized and then combined. To this purpose, these deviations are divided by their optimal value. Also, the importance of two cost-effectiveness and budget impact indicators is different and their weight needs to be added to the objective function. In other words, the significance obtained from the experts' pairwise comparisons about each index is considered as the weight of undesirable deviations in the objective function of the goal programming. Therefore, the general form of the objective function of the weighted normalized goal programming is as following:
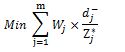
The extended model for this study is as follows:
Where,

is the optimal value of the i
th objective function when solved separately, and W
i and

are the weight of and the undesirable deviation from the j
th goal; 1 and 2 are the indexes for the first and the second objective functions. Solving this model will result in the optimal purchase amount of each technology. The optimal amount of satisfaction is also obtained from a balanced combination of satisfaction from
different indicators.
Conclusion
It can be stated that the proposed model is limited to its general concept in this paper, It means that for correction and approval of this model, it needs to be piloted by some technologies which were prepared to be used in the healthcare system; however, the authors believe that the model (combination approach of multi-attribute and multi-objective decision-making techniques) can be developed to help solve the problem of determining the number of technologies needed to implement in the health systems (in Appraisal phase or Post- Appraisal phase).
Acknowledgements
None.
Conflict of interests
Authors declared that they have no conflict of interests.
Authors’ contributions
Arman H designed and conducted research research; Mobinizadeh M and Mohamadi E developed the model for better adaptation to the healthcare systems. Arman H, Mobinizadeh M and Mohamadi E wrote the manuscript. All authors read and approved the final manuscript.
Funding
The authors didn’t receive any funding for this research.